Sliced Wasserstein cycle consistency generative adversarial networks for fault data augmentation of an industrial robot
Abstract:
We investigate the role of the loss function in cycle consistency generative adversarial networks (CycleGANs). Namely, the sliced Wasserstein distance is proposed for this type of generative model. Both the unconditional and the conditional CycleGANs with and without squeeze-and-excitation mechanisms are considered. Two data sets are used in the evaluation of the models, i.e., the well-known MNIST and a real-world in-house data set acquired for an industrial robot fault diagnosis. A comprehensive set of experiments show that, for both the unconditional and the conditional cases, sliced Wasserstein distance outperforms classic Wasserstein distance in CycleGANs. For the robot faulty data augmentation a model compatibility of 99.73% (conditional case) and 99.21% (unconditional case) were observed. In some cases, the improvement in convergence efficiency was higher than 2 (two) orders of magnitude.
Año de publicación:
2023
Keywords:
- Conditional cycle consistency generative adversarial networks
- Cycle consistency generative adversarial networks
- Scarce faulty data augmentation
- industrial robots
- Generative Adversarial Networks
- Sliced Wasserstein distance
Fuente:
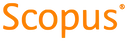
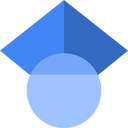
Tipo de documento:
Article
Estado:
Acceso restringido
Áreas de conocimiento:
- Inteligencia artificial
- Ciencias de la computación
Áreas temáticas:
- Métodos informáticos especiales
- Ingeniería y operaciones afines
- Ciencias de la computación