Smote-cov: A new oversampling method based on the covariance matrix
Abstract:
Nowadays, many machine learning tasks involve learning from imbalanced datasets, leading to misclassification of the minority class. One of the state-of-the-art approaches to “solve” this problem at the data level is the Synthetic Minority Oversampling Technique (SMOTE), which in turn uses KNN to select and generate new instances. However, those approaches do not take into account the attributes’ dependency relationship. This chapter presents SMOTE-Cov, a modified SMOTE that uses the Covariance Matrix instead of KNN to balance datasets, with continuous attributes and binary class. We implemented two variants SMOTE-CovI, which generates new values within the interval of each attribute, and SMOTE-CovO, which allows some values to be outside the interval of the attributes. SMOTE-Cov was validated by means of an experimental study using C4.5 as a classifier. The results show that our approach displays similar performance to the state-of-the-art approaches. After applying the statistical tests of Friedman and Holm, we did not find any big significant difference.
Año de publicación:
2020
Keywords:
- Covariance matrix
- Oversampling
- Attribute dependency
- Imbalanced datasets
Fuente:
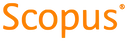
Tipo de documento:
Conference Object
Estado:
Acceso restringido
Áreas de conocimiento:
- Aprendizaje automático
- Algoritmo
- Ciencias de la computación
Áreas temáticas de Dewey:
- Ciencias de la computación

Objetivos de Desarrollo Sostenible:
- ODS 9: Industria, innovación e infraestructura
- ODS 17: Alianzas para lograr los objetivos
- ODS 8: Trabajo decente y crecimiento económico
