Solution of large-scale supply chain models using graph sampling & coarsening
Abstract:
We present a graph sampling and coarsening scheme (gSC) for computing lower and upper bounds for large-scale supply chain models. An edge sampling scheme is used to build a low-complexity problem that is used to finding an approximate (but feasible) solution for the original model and to compute a lower bound (for a maximization problem). This scheme is similar in spirit to the so-called sample average approximation scheme, which is widely used for the solution of stochastic programs. A graph coarsening (aggregation) scheme is used to compute an upper bound and to estimate the optimality gap of the approximate solution. The coarsening scheme uses node sampling to select a small set of support nodes that are used to guide node/edge aggregation and we show that the coarsened model provides a relaxation of the original model and a valid upper bound. We provide evidence that gSC can yield significant improvements in solution time and memory usage over state-of-the-art solvers. Specifically, we study a supply chain design model (a mixed-integer linear program) that contains over 38 million variables and show that gSC finds a solution with an optimality gap of <0.5% in less than 22 minutes.
Año de publicación:
2022
Keywords:
- Graph coarsening
- large-scale optimization
- supply chain
- Graph sampling
Fuente:
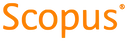
Tipo de documento:
Article
Estado:
Acceso restringido
Áreas de conocimiento:
- Logística
- Optimización matemática
- Optimización matemática
Áreas temáticas:
- Dirección general
- Ciencias de la computación
- Comercio, comunicaciones, transporte