Spatial and temporal feature extraction using a restricted boltzmann machine model
Abstract:
A restricted Boltzmann machine (RBM) is a generative neural-network model with many applications, such as, collaborative filtering, acoustic modeling, and topic modeling. An RBM lacks the capacity to retain memory, making it inappropriate for dynamic data modeling as in time-series or video analysis. In this work we address this issue by proposing the p-RBM model: a generalization of the regular RBM model capable of retaining memory of p past states. We further show how to train the p-RBM model using contrastive divergence and test our model on the problem of recognizing human actions from video data using unsupervised feature extraction. Obtained results show that the p-RBM offers promising capabilities for feature-learning in classification applications.
Año de publicación:
2018
Keywords:
- Sequential data
- Human Action Recognition
- VÍDEO
- Unsupervised feature extraction
- Neural networks
- Restricted Boltzmann machines
Fuente:
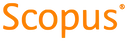
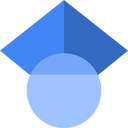
Tipo de documento:
Conference Object
Estado:
Acceso restringido
Áreas de conocimiento:
- Aprendizaje automático
- Ciencias de la computación
Áreas temáticas:
- Ciencias de la computación