Spatial estimation of chronic respiratory diseases based on machine learning procedures—an approach using remote sensing data and environmental variables in quito, Ecuador
Abstract:
Over the last few years, the use of remote sensing data in different applications such as estimation of air pollution concentration and health applications has become very popular and new. Thus, some studies have established a possible relationship between environmental variables and respiratory health parameters. This study proposes to estimate the prevalence of Chronic Respiratory Diseases, where there is a relationship between remote sensing data (Landsat 8) and environmental variables (air pollution and meteorological data) to determine the number of hospital discharges of patients with chronic respiratory diseases in Quito, Ecuador, between 2013 and 2017. The main objective of this study is to establish and evaluate an alternative LUR model that is capable of estimate the prevalence of chronic respiratory diseases, in contrast with traditional LUR models, which typically assess air pollutants. Moreover, this study also evaluates different analytic techniques (multiple linear regression, multilayer perceptron, support vector regression, and random forest regression) that often form the basis of spatial models. The results show that machine learning techniques, such as support vector machine, are the most effective in computing such models, presenting the lowest root-mean-square error (RMSE). Additionally, in this study, we show that the most significant remote sensing pbkp_redictors are the blue and infrared bands. Our proposed model is a spatial modeling approach that is capable of determining the prevalence of chronic respiratory diseases in the city of Quito, which can serve as a useful tool for health authorities in policy- and decision-making.
Año de publicación:
2020
Keywords:
- QUITO
- Respiratory disease
- Spatial models
- remote sensing
- Machine learning
Fuente:
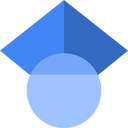
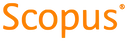
Tipo de documento:
Article
Estado:
Acceso restringido
Áreas de conocimiento:
- Salud pública
- Aprendizaje automático
- Epidemiología
Áreas temáticas de Dewey:
- Enfermedades
- Ingeniería sanitaria
- Otros problemas y servicios sociales