Stac-Da: Structure Aware Cross-Modality Domain Adaptation Framework with Image and Feature Level Adaptation for Medical Image Segmentation
Abstract:
In this work, we present an unsupervised Structure Aware Cross-modality Domain Adaptation (StAC-DA) framework for medical image segmentation. StAC-DA implements an image-and feature-level adaptation in a sequential two-step approach. The first step performs an image-level alignment, where images from the source domain are translated to the target domain by implementing a CycleGAN based model. The latter model includes a structure-aware network that preserves the shape of the anatomical structure during translation. The second step consist of a feature-level alignment. A U-Net network is trained with the transformed source domain images and target domain images in an adversarial manner to produce probable segmentations for the target domain. The proposed framework is evaluated on the problem of bidirectional cardiac substructure segmentation from the Multi-Modality Whole Heart Segmentation Challenge dataset. StAC-DA outperforms leading unsupervised domain adaptation approaches.
Año de publicación:
Keywords:
Fuente:
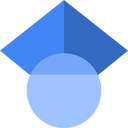
Tipo de documento:
Other
Estado:
Acceso abierto
Áreas de conocimiento:
- Aprendizaje automático
- Ciencias de la computación
- Laboratorio médico
Áreas temáticas:
- Enfermedades
- Medicina y salud