Stochastic analysis and classification of 4-area cardiac auscultation signals using Empirical Mode Decomposition and acoustic features
Abstract:
As cardiac murmurs do not generally appear in every area of auscultation, this paper presents an effective approach for cardiac murmur detection based on stochastic analysis of acoustic features derived from Empirical Mode Decomposition (EMD) and Hilbert-Huang Transform (HHT) of phonocardiographic (PCG) signals made up by the 4-Standard Auscultation Areas (SAA). The 4-SAA PCG database belongs to the National University of Colombia. Mel-Frequency Cepstral Coefficients (MFCC) and statistical moments of HHT were estimated over EMD components. An ergodic HMM was applied on the feature space, randomly initialized and trained by expectation maximization with a convergence at 10e-6 and a maximum iteration number of 1000. Global classification results for 4-SAA were around 98.7% with satisfactory sensitivity and specificity results, using a 30-fold cross-validation procedure (70/30 split). The representation capability of the EMD technique applied to 4-SAA PCG signals and stochastic analysis of acoustic features offered a high performance to detect cardiac murmurs. © 2012 CCAL.
Año de publicación:
2012
Keywords:
Fuente:
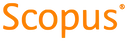
Tipo de documento:
Conference Object
Estado:
Acceso restringido
Áreas de conocimiento:
- Aprendizaje automático
- Enfermedad cardiovascular
Áreas temáticas:
- Fisiología humana