Supervised Machine Learning Applied to Non-Invasive EMG Signal Classification for an Anthropomorphic Robotic Hand
Abstract:
Advances in hardware development have created robotic hands able to mimic the appearance and functionality of the human counterpart (i.e., Anthropomorphic Robotic Hand (ARH)). Non-invasive signal identification and classification to control the robotic hands remains a challenge for real scenarios. Myoelectric sensors provide valuable information of muscle activity. However, the subject's gesture identification through myoelectric signals is a complex task. In this work, we implemented a non-invasive EMG-Based control system for an ARH based on artificial neural nets. Electromyography (EMG) data were pre-processed using filtering and scaling. Then, for each subject, 20 relevant features were extracted and selected. Next, eight machine learning architectures were used for gesture identification. Finally, the control system was implemented using custom scripts in Python, communicating with a six DoF robotic hand using an ESP32 microcontrolled card. As a result of the evaluation with the classifiers, the Multi-layer Perceptron obtained the highest accuracy of 84.78%. The implemented control system was open-loop, based on multiple inputs with a single output for each servomotor, with two seconds of delay. The EMG data classification based on artificial neural networks could be an essential resource to increase the number of independent movements performed by robotic hands.
Año de publicación:
2022
Keywords:
- EMG Machine Learning
- EMG Data Classification
- Artificial Neural Network
- Anthropomorphic robotic hand
Fuente:
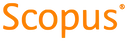
Tipo de documento:
Conference Object
Estado:
Acceso restringido
Áreas de conocimiento:
- Robótica
- Ciencias de la computación
Áreas temáticas:
- Métodos informáticos especiales
- Programación informática, programas, datos, seguridad
- Física aplicada