Supervised Statistical Learning for Cancer Detection in Dehydrated Excised Tissue with Terahertz Imaging
Abstract:
This paper proposes a new supervised image segmentation algorithm for the detection of breast cancer using terahertz (THz) imaging. Even though unsupervised learning algorithms have achieved promising results in THz image segmentation, reliable segmentation of tissues with three or more regions, such as cancer, fat and muscle, still remains a major challenge. We propose to tackle this challenge by developing a supervised statistical learning method based on multi-class Bayesian ordinal probit regression. The proposed algorithm utilizes a latent variable for the categorical classification of each pixel within the image. The model parameters are estimated through a Markov chain Monte Carlo (MCMC) process during the training phase. Experimental results in murine formalin-fixed paraffin-embedded (FFPE) breast cancer samples demonstrated that the proposed supervised model outperforms alternative unsupervised methods.
Año de publicación:
2020
Keywords:
Fuente:
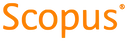
Tipo de documento:
Conference Object
Estado:
Acceso restringido
Áreas de conocimiento:
- Cáncer
- Aprendizaje automático
Áreas temáticas:
- Enfermedades
- Medicina y salud
- Ciencias de la computación