Support vector machine technique as classifier of impaired body fat percentage
Abstract:
Excess weight and obesity are indicators of an unhealthy or harmful accumulation of fat that can be dangerous to health. Body mass index (BMI) refers to height-to-weight radio and is often used to identify overweight and obesity in adults. Although BMI is commonly used to diagnose obesity and overweight, it is ineffective in differentiating between high muscle mass and elevated body fat mass. Body fat percentage (BF%) is one of the best pbkp_redictors of obesity because it quantifies adipose tissue. The Deurenberg equation is among the indirect methods to measure BF%; it uses BMI, age, and sex as parameters to calculate the BF%. Machine learning techniques demonstrated to be a good classifier of overweight, obesity, and diseases related to insulin resistance and metabolic syndrome. This study intends to evaluate anthropometric parameters as classifiers of BF% alteration using support vector machines and the Deurenberg equation for BF% estimation. The database used consisted of 1978 individuals with 24 different anthropometric measurements. The results suggest the SVM as a suitable technique for classifying individuals with normal and abnormal BF% values. Accuracy, F1 score, PPV, NPV, and sensitivity were above 0.8. Besides, the specificity value is below 0.7, which indicates that false positives may occur. As future work, this research intends to apply neural networks as a classification technique.
Año de publicación:
2021
Keywords:
- Support Vector Machine
- anthropometric measurements
- Fat body percentage
- Monte Carlo cross validation
Fuente:
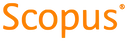
Tipo de documento:
Conference Object
Estado:
Acceso abierto
Áreas de conocimiento:
- Aprendizaje automático
Áreas temáticas:
- Farmacología y terapéutica
- Interacción social
- Fisiología humana