Support vector machine-based approach for multi-labelers problems
Abstract:
We propose a first approach to quantify the panelist's labeling generalizing a soft-margin support vector machine classifier to multi-labeler analysis. Our approach consists of formulating a quadratic optimization problem instead of using a heuristic search algorithm. We determine penalty factors for each panelist by incorporating a linear combination in the primal formulation. Solution is obtained on a dual formulation using quadratic programming. For experiments, the well-known Iris with multiple simulated artificial labels and a multi-label speech database are employed. Obtained penalty factors are compared with both standard supervised and non-supervised measurements. Promising results show that proposed method is able to asses the concordance among panelists considering the structure of data.
Año de publicación:
2013
Keywords:
Fuente:
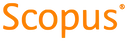
Tipo de documento:
Conference Object
Estado:
Acceso restringido
Áreas de conocimiento:
- Aprendizaje automático
- Algoritmo
- Ciencias de la computación
Áreas temáticas:
- Programación informática, programas, datos, seguridad