Support vector machines for interval discriminant analysis
Abstract:
The use of data represented by intervals can be caused by imprecision in the input information, incompleteness in patterns, discretization procedures, prior knowledge insertion or speed-up learning. All the existing support vector machine (SVM) approaches working on interval data use local kernels based on a certain distance between intervals, either by combining the interval distance with a kernel or by explicitly defining an interval kernel. This article introduces a new procedure for the linearly separable case, derived from convex optimization theory, inserting information directly into the standard SVM in the form of intervals, without taking any particular distance into consideration. © 2008 Elsevier B.V. All rights reserved.
Año de publicación:
2008
Keywords:
- classification
- Convex optimization
- Kernel machines
- interval analysis
Fuente:
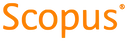
Tipo de documento:
Article
Estado:
Acceso restringido
Áreas de conocimiento:
- Aprendizaje automático
- Algoritmo
Áreas temáticas de Dewey:
- Programación informática, programas, datos, seguridad
- Métodos informáticos especiales
- Funcionamiento de bibliotecas y archivos

Objetivos de Desarrollo Sostenible:
- ODS 9: Industria, innovación e infraestructura
- ODS 17: Alianzas para lograr los objetivos
- ODS 8: Trabajo decente y crecimiento económico
