Target detection using radar processors based on machine learning
Abstract:
Target detection is one of the most important applications of radar systems. However, the task of processing echoed signals to determine whether a valid target exists, or if it is just noise, is not simple. Constant false alarm rate detectors have traditionally been used in radar processors using classical hypothesis testing methods, and moving target indicator (MTI) or moving target detector (MTD) algorithms to discriminate targets from clutter (i.e., unwanted noise). On the other hand, machine learning research has significantly improved the performance and application of classifiers that I earn by example. Thus, this paper evaluates the implementation of target detection using radar processors based on machine learning classifiers, namely random decision forests and recurrent neural networks (deep learning). The experimental comparison implemented in this work is based on a testbed that replicates the configuration of the Oerlikon Skyguard surveillance radar. Obtained results are also compared with advanced MTI/MTD detectors, showing that machine learning algorithms discriminate targets from clutter with remarkable precision. In particular, deep learning helps to improve radar detection rates at very low signal-to-clutter ratios mainly, keeping false alarm rates under predetermined values.
Año de publicación:
2020
Keywords:
- Radar detection
- Radar clutter
- Random decision forest
- Oerlikon Skyguard radar
- Recurrent neural networks
Fuente:
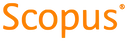
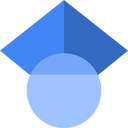
Tipo de documento:
Conference Object
Estado:
Acceso restringido
Áreas de conocimiento:
- Aprendizaje automático
- Ciencias de la computación
Áreas temáticas:
- Ciencias de la computación
- Física aplicada
- Métodos informáticos especiales