Teacher-Student Semi-supervised Approach for Medical Image Segmentation
Abstract:
Accurate segmentation of anatomical structures is a critical step for medical image analysis. Deep learning architectures have become the state-of-the-art models for automatic medical image segmentation. However, these models require an extensive labelled dataset to achieve a high performance. Given that obtaining annotated medical datasets is very expensive, in this work we present a two-phase teacher-student approach for semi-supervised learning. In phase 1, a three network U-Net ensemble, denominated the teacher, is trained using the labelled dataset. In phase 2, a student U-Net network is trained with the labelled dataset and the unlabelled dataset with pseudo-labels produced with the teacher network. The student network is then used for inference of the testing images. The proposed approach is evaluated on the task of abdominal segmentation from the FLARE2022 challenge, achieving a mean 0.53 dice, 0.57 NSD, and 44.97 pbkp_rediction time on the validation set.
Año de publicación:
2022
Keywords:
- semi-supervised learning
- image segmentation
- Medical image analysis
Fuente:
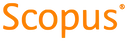
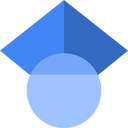
Tipo de documento:
Conference Object
Estado:
Acceso restringido
Áreas de conocimiento:
- Laboratorio médico
- Visión por computadora
- Ciencias de la computación
Áreas temáticas:
- Medicina y salud
- Farmacología y terapéutica
- Funcionamiento de bibliotecas y archivos