Temporal validated meta-learning for long-term forecasting of chaotic time series using Monte Carlo cross-validation
Abstract:
Forecasting long-term values of chaotic time series is a difficult task, but it is required in several domains such as economy, medicine and astronomy. State-of-the-art works agree that the best accuracy can be obtained combining forecasting models. However, selecting the appropriate models and the best way to combine them is an open problem. Some researchers have been focusing on using prior knowledge of the performance of the models for combining them. A way to do so is by meta-learning, which is the process of automatically learning from tasks and models showing the best performances. Nevertheless, meta-learning in time series impose no trivial challenges; some requirements are to search the best model, to validate estimations, and even to develop new meta-learning methods. The new methods would consider performance variances of the models over time. This research addresses the meta-learning problem of how to select and combine models using different parts of the pbkp_rediction horizon. Our strategy, called ‘‘Temporal Validated Combination’’ (TVC), consists of splitting the pbkp_rediction horizon into three parts: short, medium and long-term windows. Next, for each window, we extract knowledge about what model has the best performance. This knowledge extraction uses a Monte Carlo cross-validation process. Using this, we are able to improve the long-term pbkp_rediction using different models for each pbkp_rediction window. The results reported in this chapter show that TVC obtained an average improvement of 1% in the pbkp_rediction of 56 points of the NN5 time series, when compared to a combination of best models based on a simple average. NARX neural networks and ARIMA models were used for building the pbkp_redictors and the SMAPE metric was used for measuring performances.
Año de publicación:
2014
Keywords:
Fuente:
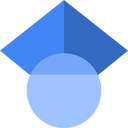
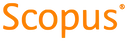
Tipo de documento:
Article
Estado:
Acceso restringido
Áreas de conocimiento:
- Aprendizaje automático
- Optimización matemática
- Serie temporal
Áreas temáticas:
- Sistemas