The Concatenated Dynamic Convolutional and Sparse Coding on Image Artifacts Reduction
Abstract:
In order to enhance compressed JPEG image, a deep convolutional sparse coding network is proposed in this article. The network integrates state-of-the-art dynamic convolution to extract multi-scale image features, and uses convolutional sparse coding to separate image artifacts to generate coded feature for the final image reconstruction. Since this architecture consolidates model-based convolutional sparse coding with deep neural network, that allow this method has more interpretability. Also, compared with the existing network, which uses a dilated convolution as a feature extraction approach, this proposed concatenated dynamic method has improved de-blocking result in both numerical experiments and visual effect. Besides, in the higher compressed quality task, the proposed model has more pronounced improvement in reconstructed image quality evaluations.
Año de publicación:
2021
Keywords:
- image reconstruction
- Dynamic convolution
- deep learning
- Sparse coding
Fuente:
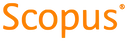
Tipo de documento:
Conference Object
Estado:
Acceso restringido
Áreas de conocimiento:
- Visión por computadora
- Ciencias de la computación
- Computadora
Áreas temáticas:
- Métodos informáticos especiales