The Impact of Content Deletion on Tabular Data Similarity Using Contextual Word Embeddings
Abstract:
Table retrieval is the task of answering a search query with a ranked list of tables that are considered as relevant to that query. Computing table similarity is a critical part of this process. Current Transformer-based language models have been successfully used to obtain word embedding representations of the tables to calculate their semantic similarity. Unfortunately, obtaining word embedding representations of large tables with thousands or millions of rows can be a computationally expensive process. The present work states the hypothesis that much of the content of a table can be deleted (i.e. rows can be dropped) without significantly affecting its word embedding representation, thus maintaining system performance at a much lower computational cost. To test this hypothesis a study was carried out using two different datasets and three state-of-the-art language models. The results obtained reveal that, in large tables, keeping just 10% of the content produces a word embedding representation that is 90% similar to the original one.
Año de publicación:
2023
Keywords:
Fuente:
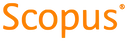
Tipo de documento:
Conference Object
Estado:
Acceso restringido
Áreas de conocimiento:
- Aprendizaje automático
- Ciencias de la computación
- Ciencias de la computación
Áreas temáticas:
- Programación informática, programas, datos, seguridad
- Métodos informáticos especiales
- Biblioteconomía y Documentación informatica