Time Series Forecasting with Quantum Machine Learning Architectures
Abstract:
Time series forecasting has been a topic of special interest due to its applications in Finance, Physics, Environmental Sciences and many other fields. In this article, we propose two classical-quantum hybrid architectures for time series forecasting with a multilayered structure inspired by the multilayer perceptron (MLP): a Quantum Neural Network (QNN) and a Hybrid-Quantum Neural Network (HQNN). These architectures incorporate quantum variational circuits with specific encoding schemes and the optimization is carried out by a classical computer. The performance of the proposed hybrid models is evaluated in four forecasting problems: Mackey-Glass time series and USD-to-euro currency exchange rate forecasting (univariate time series) as well as Lorenz attractor and pbkp_rediction of the Box-Jenkins (Gas Furnace) time series (multivariate time series). The experiments were conducted by using the built-in Pennylane simulator lighting.qubit and Pytorch. Finally, these architectures, compared to the MLP, CNN and LSTM show a competitive performance with a similar number of trainable parameters.
Año de publicación:
2022
Keywords:
- Quantum machine learning
- Time series forecasting
- Quantum neural networks
Fuente:
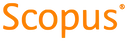
Tipo de documento:
Conference Object
Estado:
Acceso restringido
Áreas de conocimiento:
- Aprendizaje automático
- Ciencias de la computación
Áreas temáticas:
- Ciencias de la computación
- Programación informática, programas, datos, seguridad
- Métodos informáticos especiales