TnTViT-G: Transformer in Transformer Network for Guidance Super Resolution
Abstract:
Image Super Resolution is a potential approach that can improve the image quality of low-resolution optical sensors, leading to improved performance in various industrial applications. It is important to emphasize that most state-of-the-art super resolution algorithms often use a single channel of input data for training and inference. However, this practice ignores the fact that the cost of acquiring high-resolution images in various spectral domains can differ a lot from one another. In this paper, we attempt to exploit complementary information from a low-cost channel (visible image) to increase the image quality of an expensive channel (infrared image). We propose a dual stream Transformer-based super resolution approach that uses the visible image as a guide to super-resolve another spectral band image. To this end, we introduce Transformer in Transformer network for Guidance super resolution, named TnTViT-G, an efficient and effective method that extracts the features of input images via different streams and fuses them together at various stages. In addition, unlike other guidance super resolution approaches, TnTViT-G is not limited to a fixed upsample size and it can generate super-resolved images of any size. Extensive experiments on various datasets show that the proposed model outperforms other state-of-the-art super resolution approaches. TnTViT-G surpasses state-of-the-art methods by up to 0.19 sim 2.3dB , while it is memory efficient.
Año de publicación:
2023
Keywords:
- guidance super resolution
- Single image super resolution
- TRANSFORMERS
- Convolutional neural network
Fuente:
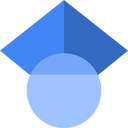
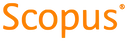
Tipo de documento:
Article
Estado:
Acceso abierto
Áreas de conocimiento:
- Aprendizaje automático
- Software
Áreas temáticas:
- Ciencias de la computación