Topic Modeling for Automatically Identification of STEM Barriers
Abstract:
Topic modeling allows identifying topics automatically from a set of documents. Latent Dirichlet Allocation (LDA) is an algorithm widely used to perform topic modeling. This research applied LDA to identify topics from digital scientific articles that face barriers in STEM fields. Since gender imbalances unfavourable for women have been demonstrated in numerous studies, we created a corpus based on abstracts of papers indexed by Scopus and published between 2000 to 2020. To address the search, we used some keywords related to STEM, women, barriers and gender; as a result, we collected 141 abstracts of digital articles. Then, we apply some techniques to prepare the text and create a computable representation of each abstract. After classifying the data and finding the most important topics, the LDA learning algorithm analysed the dataset. Finally, to identify the best experiment, we used the values of coherence and the distance inter-topic. Results reveal that discovered topics are related to gender differences that make women gaps in STEM careers and works, initiatives to improve gender diversity in STEM faculties, barriers and gender differences in areas like software and industry, factors that could favour women access to STEM disciplines and models or programs to improve the access of women STEM fields.
Año de publicación:
2022
Keywords:
- STEM
- Lda
- Women
- Topic modeling
Fuente:
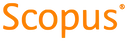
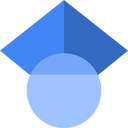
Tipo de documento:
Conference Object
Estado:
Acceso restringido
Áreas de conocimiento:
- Análisis de datos
Áreas temáticas:
- Filosofía y teoría
- Programación informática, programas, datos, seguridad
- Funcionamiento de bibliotecas y archivos