Toward a Social Media Emergency Events Detection Based on Supervised Learning
Abstract:
Urban emergency events affect many lives and property of people every day. They should be tackled in a fast, precise, and with an early detection goal. In recent years, social media has become popular, turning its users into proper “citizen sensors,” which means a person who, through devices, records incidents on these platforms. Therefore, they become real-time communicators of emergency events. This paper presents a preliminary study based on supervised learning toward social media emergency events detection. This approach has four phases: the first two correspond to data acquisition and preprocessing, where tweets available in a public repository about emergency and non-emergency were taken, filtered, and converted through a process of lemma feature extraction. In the third phase, four most commonly used supervised algorithms were implemented to detect tweets containing emergency events. Finally, using validation metrics and algorithms to detect emergency and non-emergency reported in the tweets was verified. Computational results showed that all evaluated algorithms achieved average rates of 85.38% of accuracy meanwhile that in execution time about 23 seconds, to detect a real emergency event. The best algorithm when trade-offs between execution time and emergency detection capability are considered was the SVM.
Año de publicación:
2022
Keywords:
- Emergency events
- classification
- Emergency detection
- Social media
Fuente:
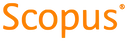
Tipo de documento:
Conference Object
Estado:
Acceso restringido
Áreas de conocimiento:
- Redes sociales
- Aprendizaje automático
- Ciencias de la computación
Áreas temáticas:
- Funcionamiento de bibliotecas y archivos
- Otros problemas y servicios sociales
- Lengua