Towards adaptive weight vectors for multiobjective evolutionary algorithm based on decomposition
Abstract:
The decomposition method in multiobjective evolutionary algorithms (MOEA/D) is an effective approach to evolve solutions along predefined weight vectors for solving multiobjective optimization problems (MOPs). However, obtaining evenly distributed weight vectors for different types of MOPs is a challenge problem especially when the true Pareto fronts (PFs) are unknown before a MOEA/D starts. In this paper, a new MOEA/D with a fast hypervolume archive (called FV-MOEA/D) is proposed to adaptively adjust the weight vectors for various shapes of PFs. The core idea of FV-MOEA/D is to periodically adjust weight vectors based on solutions in the proposed archive, in which convergence and diversity are maintained by maximizing hypervolume. Experimental studies on 58 benchmark MOPs in jMetal demonstrate that the proposed FV-MOEA/D not only reached higher hypervolumes when compare to five classical MOEAs i.e., NSGAII, SPEA2, IBEA, FV-MOEA and MOEA/D, but also obtained well distributed weight vectors on PFs with different geometrical characteristics.
Año de publicación:
2016
Keywords:
Fuente:
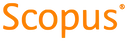
Tipo de documento:
Conference Object
Estado:
Acceso restringido
Áreas de conocimiento:
- Evolución
- Algoritmo
Áreas temáticas:
- Programación informática, programas, datos, seguridad