Tree-based pbkp_rediction on incomplete data using imputation or surrogate decisions
Abstract:
The goal is to investigate the pbkp_rediction performance of tree-based techniques when the available training data contains features with missing values. Also the future test cases may contain missing values and thus the methods should be able to generate pbkp_redictions for such test cases. The missing values are handled either by using surrogate decisions within the trees or by the combination of an imputation method with a tree-based method. Missing values generated according to missing completely at random (MCAR), missing at random (MAR) and missing not at random (MNAR) mechanisms are considered with various fractions of missing data. Imputation models are built in the learning phase and do not make use of the response variable, so that the resulting procedures allow to pbkp_redict individual incomplete test cases. In the empirical comparison, both classification and regression problems are considered using a simulated and real-life datasets. The performance is evaluated by misclassification rate of pbkp_redictions and mean squared pbkp_rediction error, respectively. Overall, our results show that for smaller fractions of missing data an ensemble method combined with surrogates or single imputation suffices. For moderate to large fractions of missing values ensemble methods based on conditional inference trees combined with multiple imputation show the best performance, while conditional bagging using surrogates is a good alternative for high-dimensional pbkp_rediction problems. Theoretical results confirm the potential better pbkp_rediction performance of multiple imputation ensembles.
Año de publicación:
2015
Keywords:
- Multiple imputation
- Conditional inference tree
- pbkp_rediction
- Surrogate decision
- Missing data
Fuente:
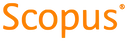
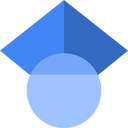
Tipo de documento:
Article
Estado:
Acceso restringido
Áreas de conocimiento:
- Aprendizaje automático
- Ciencias de la computación
Áreas temáticas:
- Programación informática, programas, datos, seguridad
- Métodos informáticos especiales
- Funcionamiento de bibliotecas y archivos