Two novel clustering performance measures based on coherence and relative assignments of clusters
Abstract:
This work proposes two novel alternatives for dealing with the highly important issue of the clustering performance estimation. One of the measures is the cluster coherence aimed to quantifying the normalized ratio of cuts within a graph-partitioning framework, and therefore it uses a graph-driven approach to explore the nature of data regarding the cluster assignment. The another one is the probability-based-performance quantifier, which calculates a probability value for each cluster through relative frequencies. Proposed measures are tested on some clustering representative techniques applied to real and artificial data sets. Experimental results probe the readability and robustness to noisy labels of our measures.
Año de publicación:
2017
Keywords:
- probabilities
- Cluster coherence
- Graph-partitioning
- Relative frequencies
- Clustering
Fuente:
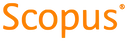
Tipo de documento:
Conference Object
Estado:
Acceso restringido
Áreas de conocimiento:
- Aprendizaje automático
- Ciencias de la computación
- Estadísticas
Áreas temáticas de Dewey:
- Programación informática, programas, datos, seguridad

Objetivos de Desarrollo Sostenible:
- ODS 9: Industria, innovación e infraestructura
- ODS 17: Alianzas para lograr los objetivos
- ODS 4: Educación de calidad
