A data analytics method based on data science and machine learning for bank risk pbkp_rediction in cbkp_redit applications for financial institutions
Abstract:
Nowadays, banks grant cbkp_redits so that customers can acquire a good or service, start or improve a business, among other benefits. The problems that may arise are over-indebtedness and low saving possibilities on the part of customers, so the tendency is the risk of default. Financial institutions require tools for default risk analysis and problem pbkp_rediction. Therefore, in this research, a data analysis method based on data science and machine learning is proposed for bank risk pbkp_rediction in cbkp_redit applications for financial institutions. For the analysis process and for the pbkp_rediction of a cbkp_redit, pbkp_redictive analysis methods are used: Genetic Algorithms (GA), Random Forest (RF), K-Nearest-Neighbor (KNN), Support Vector Machines (SVM) and Neural Network (NN). Quality metrics such as Accuracy, Precision, Recall and F1 Score are used to evaluate the results. A public dataset called Statlog [1] is used. This work opens the door for data analysis in different banking services. The main objective of this research is to help financial companies to optimize their processes.
Año de publicación:
2022
Keywords:
Fuente:
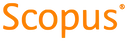
Tipo de documento:
Conference Object
Estado:
Acceso restringido
Áreas de conocimiento:
- Análisis de datos
- Finanzas
- Ciencias de la computación
Áreas temáticas:
- Programación informática, programas, datos, seguridad
- Economía
- Métodos informáticos especiales