U-Net Variations for Spontaneous Intracranial Hemorrhages Detection on CT Images
Abstract:
Brain injuries represent one of the most severe medical problems that affect people health worldwide. These injuries are classified in different types, being the intracranial hemorrhages (ICH) the most critical. A delayed treatment of ICH may lead to irreparable brain damage such as permanent disability, significant patient quality-of-life change, and even worse, death. Brain computed tomography (CT) examination is the preferred method to diagnose ICH. However, manually reading brain CT images can be time-consuming, delayed, and prone to human error, which may worsen the patients' health. In this context, a new intracranial hemorrhages detector based on a deep CNN architecture that automatically identifies and segments ICH in CT scan images is proposed. Based on the standard U-Net, two architectures named, Unet1 and Unet2, have been developed and validated on a public database. The Unet2 model trained with 1,000 epochs and a batch size of 32 obtained the best results. The mean of interception over union score of 0.85 and Dice coefficient of 0.89 during the training stage revealed a capacity to successfully segment more than the 85% of the lesion area. Additionally, the model was tested with unseen images in training and obtained a mean of interception over union and dice coefficient scores of 0.86 and 0.91, respectively. The latter results demonstrate the generalization strength of the model to accurately identify and segment the lesion area and the suitability to be implemented in the final ICH detector.
Año de publicación:
2022
Keywords:
- Convolutional neural network
- Computerized tomography scan
- image segmentation
- deep learning
- ICH
- U-net
Fuente:
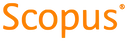
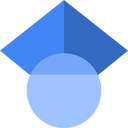
Tipo de documento:
Conference Object
Estado:
Acceso restringido
Áreas de conocimiento:
- Neurología
Áreas temáticas:
- Ginecología, obstetricia, pediatría, geriatría
- Enfermedades
- Medicina y salud