Unsupervised classification system for arrhythmogenic sources detection from electrograms. A simulation study
Abstract:
The CFAEs are widely reported as targeted areas for ablation in attempts to stop the atrial fibrillation. This paper presents a system for detection of CFAE's areas based on unsupervised machines learning K-means and self-organized maps (SOM) over an electrogram signals provided by a 2D model of atrial fibrillation on simulated human atrial tissue. The signals were characterized using statistics measurements (mean, deviation, variance, kurtosis and skewness), Shannon's entropy and log energy entropy over the time and frequency domain. A rough set feature selection algorithm was applied to identify the more relevant features in order to classify the signals using K-means and SOM with several different cluster numbers. The performance of the classifiers was evaluated as the average distance from the elements that compound each cluster to the rotor tip of the 2D model. The best performance obtained was 2.4 ± 1 mm based on k-means algorithm with 9 clusters and using mean on time domain and instantaneous frequency kurtosis as features. This approach offered a good capability CFAE's areas identification.
Año de publicación:
2014
Keywords:
- Unsupervised Learning.
- Arrhythmogenic Sources
- electrogram
- Signal processing
Fuente:
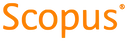
Tipo de documento:
Conference Object
Estado:
Acceso restringido
Áreas de conocimiento:
- Aprendizaje automático
- Simulación por computadora
Áreas temáticas:
- Física aplicada
- Enfermedades
- Medicina y salud