Unsupervised learning by cluster quality optimization
Abstract:
Most clustering algorithms are designed to minimize a distortion measure which quantifies how far the elements of the clusters are from their respective centroids. The assessment of the results is often carried out with the help of cluster quality measures which take into account the compactness and separation of the clusters. However, these measures are not amenable to optimization because they are not differentiable with respect to the centroids even for a given set of clusters. Here we propose a differentiable cluster quality measure, and an associated clustering algorithm to optimize it. It turns out that the standard k-means algorithm is a special case of our method. Experimental results are reported with both synthetic and real datasets, which demonstrate the performance of our approach with respect to several standard quantitative measures.
Año de publicación:
2018
Keywords:
- K-Means
- unsupervised learning
- Cluster quality measures
- Clustering
Fuente:
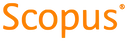
Tipo de documento:
Article
Estado:
Acceso restringido
Áreas de conocimiento:
- Aprendizaje automático
- Algoritmo
- Algoritmo
Áreas temáticas:
- Métodos informáticos especiales
- Programación informática, programas, datos, seguridad
- Funcionamiento de bibliotecas y archivos