Unsupervised learning for the droplet evolution pbkp_rediction and process dynamics understanding in inkjet printing
Abstract:
Droplet jetting behavior largely determines the final drop deposition quality in the inkjet printing process. Forming such behavior is governed by the fluid flow pattern. Therefore, a measurement of the flow pattern is of great importance for improving the printing quality of the inkjet printing process. Most of the current works use static images for the study of the drop evolution process. The problem of the static images is that the images cannot recognize the motion information (i.e., temporal transformation) of the droplet. Thus the information of the jetting process in the temporal domain will be lost. Instead of using the images, this paper takes the video data as the study subject to investigate the droplet evolution behavior in the inkjet printing process. Moreover, this paper introduces a deep learning method for the study of such video data. Compared to most of the current learning approaches conducted in a supervised/semi-supervised manner for manufacturing process data, we propose an unsupervised learning method for studying the flow pattern of the droplet, which does not require well-defined ground-truth labels. Regarding the spatial and temporal transformation of the droplet in video data, we apply a deep recurrent neural network (DRNN) to implement the proposed unsupervised learning. To verify the hypothesis that the proposed method can learn a latent representation for reproducing original data, the proposed DRNN is trained and tested on both simulation and experimental datasets. Experimental results demonstrate that the proposed method can learn latent representations of the droplet jetting process video data, which is very useful for the pbkp_rediction of the droplet behavior. Furthermore, through latent space decoding, the learned representations can infer the droplet forming stimulus parameters such as material properties, which would be very helpful for further understanding of the process dynamics and achieving real-time in-situ droplet deposition quality monitoring and control.
Año de publicación:
2020
Keywords:
- Deep recurrent neural network (DRNN)
- Inkjet printing
- Video pbkp_rediction
- unsupervised learning
- Latent space decoding
Fuente:
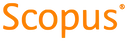
Tipo de documento:
Article
Estado:
Acceso abierto
Áreas de conocimiento:
- Aprendizaje automático
- Ciencias de la computación
Áreas temáticas:
- Programación informática, programas, datos, seguridad
- Métodos informáticos especiales
- Imprenta y actividades conexas