Use of classification trees and rule-based models to optimize the funding assignment to research projects: A case study of UTPL
Abstract:
In the process of funding research projects, two important factors must be studied. First, experts judges the potential value of a project. Secondly, the research ability is judged by the applicants previous research activity. The most appropriate way to assign the appropriate amount of money to project proposals is always a difficult decision. This work focuses on the second factor based on classifying the researchers previous research activity on an automated logical classification (accepted, rejected) resolving conflicts of interests between administration and applicants and helping in the decision-making process. As the class in these kinds of studies is usually unbalanced, because there are fewer accepted projects than rejected projects, how the use of an imbalanced dataset or a balanced dataset affects to the models is investigated by using several resampling methods. Later, several trees and rule-based machine learning techniques are used to create classification models. This is based on information from the faculty members information of the "Technical Particular University of Loja (UTPL),"in cases, with balanced datasets and those with unbalanced datasets. Multivariate analysis, feature selection, algorithm parameter tuning and validation methods are used to achieve robust classification models. The most accurate results are obtained with a rules-based model and use of the C5.0 algorithm. As the latter provides acceptable accuracy, close to 95 % when pbkp_redicting both classes and to 99 % when pbkp_redicting the accepted projects class, both the methodology and final model are validated.
Año de publicación:
2021
Keywords:
- Unbalanced dataset
- Automated logical classification
- Resampling methods
- Model validation methodology
- Funding assignment
Fuente:
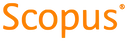
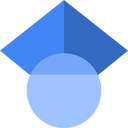
Tipo de documento:
Article
Estado:
Acceso restringido
Áreas de conocimiento:
- Análisis de datos
- Toma de decisiones
- Optimización matemática
Áreas temáticas:
- Funcionamiento de bibliotecas y archivos