Use of contextualized attention metadata for ranking and recommending learning objects
Abstract:
The tools used to search and find Learning Objects in different systems do not provide a meaningful and scalable way to rank or recommend learning material. This work propose and detail the use of Contextual Attention Metadata, gathered from the different tools used in the lifecycle of the Learning Object, to create ranking and recommending metrics to improve the user experience. Four types of metrics are detailed: Link Analysis Ranking, Similarity Recommendation, Personalized Ranking and Contextual Recommendation. While designed for Learning Objects, it is shown that these metrics could also be applied to rank and recommend other types of reusable components like software libraries. © 2006 ACM.
Año de publicación:
2006
Keywords:
- RANKING
- Attention metadata
- Recommending
- Learning objects
Fuente:
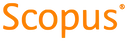
Tipo de documento:
Conference Object
Estado:
Acceso restringido
Áreas de conocimiento:
- Tecnología educativa
- Ciencias de la computación
Áreas temáticas:
- Funcionamiento de bibliotecas y archivos