Using a combination of artificial neural networks for the diagnosis of multiple sclerosis
Abstract:
Very often the number of data available in the average clinical study of a disease is small. This is one of the main obstacles in the application of neural networks to the classification of biological signals used for diagnosing diseases. A rule of thumb states that the number of parameters (weights) that can be used for training a neural network should be around 15% of the available data, to avoid overlearning. This condition puts a limit on the dimension of the input space In this paper we work with the Radial Basis Function and Functional Link artificial neural networks. To have enough data to train both neural networks, we increment the number of training elements, using randomly expanded training sets. This way the number of original signals does not constraint the dimension of the input sets. Once the radial basis function has been trained, we train four functional link neural networks using samples of positives, false positives, negatives and false negatives results of the previous one. We then test the Radial Basis Function neural network by itself, and the chain of networks. A comparison with results obtained using other methods is presented.
Año de publicación:
2011
Keywords:
- Radial Basis Functions
- Signal processing
- Health Sciences
- wavelets
- Neural networks
- Multiple Sclerosis
- Functional link architecture
Fuente:
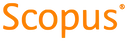
Tipo de documento:
Conference Object
Estado:
Acceso restringido
Áreas de conocimiento:
- Inteligencia artificial
- Ciencias de la computación
Áreas temáticas de Dewey:
- Enfermedades
- Medicina y salud
- Física aplicada

Objetivos de Desarrollo Sostenible:
- ODS 3: Salud y bienestar
- ODS 17: Alianzas para lograr los objetivos
- ODS 9: Industria, innovación e infraestructura
