A decoupled data-driven strategy for estimating parameters with nonlinear dependence
Abstract:
In this work, we introduce a decoupled data-driven methodology for the online estimation of parameters with nonlinear dependence. Common estimation methods for nonlinear systems rely on the incorporation of the mathematical model under study to the estimation structure. Other variational approaches trend to be more computationally expensive. In contrast, data-driven estimation methods, based on the retrospective cost model refinement (RCMR) algorithm, have demonstrated an efficient and adequate ability in the estimation of parameters. However, a better performance is observed when the estimation structure is divided into several independent sub-structures. The proposed decoupled RCMR strategy is explained and compared with the original structure using numerical examples. The results of the numerical examples show that the proposed strategy exhibits a good trade-off between the velocity of convergence and error minimization.
Año de publicación:
2020
Keywords:
- Retrospective cost model refinement
- Data-driven parameter estimation
- nonlinear parameter estimation
Fuente:
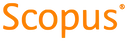
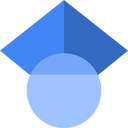
Tipo de documento:
Conference Object
Estado:
Acceso restringido
Áreas de conocimiento:
- Optimización matemática
- Sistema no lineal
- Optimización matemática
Áreas temáticas:
- Ciencias de la computación