Using an artificial neural network to improve the transformation of coordinates between classical geodetic reference frames
Abstract:
Advances in technology have allowed for the improvement of geodetic reference systems (GRSs). Relating different GRSs can be done by employing transformation parameters which may not, however, be satisfactory in certain applications due to the heterogeneous and local character of deformations caused by the procedures adopted in classical networks. Classical networks were established basically by procedures of triangulation and traverse survey, and the existence of these deformations justifies the search for new transformation methodologies. This study evaluated artificial neural networks (ANNs) as a tool for the transformation between GRSs. Frames points with known geodetic coordinates (latitude and longitude) in the South American Datum of 1969 (SAD69) system and in the older Córrego Alegre system, both still in use in Brazil, were chosen for this study. The SAD69 coordinates of the frame points were transformed into Córrego Alegre coordinates and then the computed coordinates were compared with known ones. Four tests were carried out in order to transform the coordinates. The first test involved the use of official transformation parameters and the formulas of Molodensky. In the second test, new transformation parameters were employed. In the third test new regional transformation parameters were determined, while the fourth test employed an ANN to pbkp_redict the Córrego Alegre coordinates of the check points. Results indicated that the employment of an ANN transformed the coordinates most accurately, and indicated that they can be useful in modeling deformations in classical networks. © 2007 Elsevier Ltd. All rights reserved.
Año de publicación:
2008
Keywords:
- Coordinates transformation
- artificial neural networks
- Radial basis functions reference frames
- Learning
Fuente:
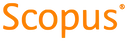
Tipo de documento:
Review
Estado:
Acceso restringido
Áreas de conocimiento:
- Aprendizaje automático
Áreas temáticas de Dewey:
- Ciencias de la computación
- Ciencias de la tierra
- Física aplicada