A deep architecture for visually analyze Pap cells
Abstract:
This work proposes a deep ANN architecture which accomplishes the reliable visual classification of abnormal Pap smear cell. The system is driven by independent agents where the first agent consists of a three layer ANN pretrained to closely track a reticle pattern. This net participates in a local close loop that oscillates and produces unique time-space versions of the visual data. This information is stabilized and sparsed in order to obtain compact data representations, with implicit space time content. The obtained representations are delivered to second level agents, formed by independent three layers ANNs dedicated to learning and recognition activities. To train the system a noise-balanced algorithm is employed, where the training set is composed by pap cells and white noise. This combination operating on finite databases and in a self controlled learning loop, auto develops enough cell recognition knowledge as to classify whole classes of Pap smear cells. The system has been tested in real time utilizing documented data bases.
Año de publicación:
2015
Keywords:
- Pap cell recognition
- deep learning
- noise balanced learning
- deep architectures
Fuente:
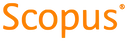
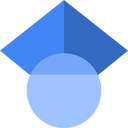
Tipo de documento:
Conference Object
Estado:
Acceso restringido
Áreas de conocimiento:
- Visión por computadora
- Ciencias de la computación
Áreas temáticas:
- Enfermedades
- Métodos informáticos especiales
- Medicina y salud