Utilizing decision tree-based thresholds in reconstructing training datasets for single-classification binary support vector machine problem from point cloud data
Abstract:
In some cases of agricultural features extraction, the binary Support Vector Machine (SVM) classification problem only requires the objective of mapping a single class across a relatively large geographic area without the need to identify other land use and land cover classes. While the conduct of ground trothing to collect field data samples is a requirement to achieve high accuracy levels, sometimes it is impractical considering the labor and cost of conducting field surveys that necessitates single species only. As such, the Cocos Nucifera or coconut, which is an agricultural class that is visually discernible through LiDAR-derived Canopy Height Model (CHM), may be classified without the need for in situ data collection. This is done by exploiting the threshold values calculated via Decision Tree (DT) algorithm in reconstructing datasets. In comparison, classification outputs from in situ training samples and from the DT-derived samples achieve similar accuracy levels, hence this study introduces a classification methodology that eliminates the need for field data gathering and manual 'training data' selection in mapping coconut species. A single-classification binary SVM has been implemented using LiDAR-derived CHM, utilizing only the elevation information contained in the point cloud data, with a grid resolution of one meter. The results suggest that automatic selection of samples is tolerable given that a representative calibration site is identified.
Año de publicación:
2017
Keywords:
- Support Vector Machine
- decision tree
- Lidar
- Cocos nucifera
- Canopy height model
Fuente:
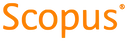
Tipo de documento:
Conference Object
Estado:
Acceso restringido
Áreas de conocimiento:
- Aprendizaje automático
- Ciencias de la computación
- Ciencias de la computación
Áreas temáticas:
- Ciencias de la computación