Very short term irradiance forecasting using the lasso
Abstract:
We find an application of the lasso (least absolute shrinkage and selection operator) in sub-5-min solar irradiance forecasting using a monitoring network. Lasso is a variable shrinkage and selection method for linear regression. In addition to the sum of squares error minimization, it considers the sum of ℓ1-norms of the regression coefficients as penalty. This bias-variance trade-off very often leads to better pbkp_redictions.One second irradiance time series data are collected using a dense monitoring network in Oahu, Hawaii. As clouds propagate over the network, highly correlated lagged time series can be observed among station pairs. Lasso is used to automatically shrink and select the most appropriate lagged time series for regression. Since only lagged time series are used as pbkp_redictors, the regression provides true out-of-sample forecasts. It is found that the proposed model outperforms univariate time series models and ordinary least squares regression significantly, especially when training data are few and pbkp_redictors are many. Very short-term irradiance forecasting is useful in managing the variability within a central PV power plant.
Año de publicación:
2015
Keywords:
- Parameter shrinkage
- Irradiance forecasting
- Monitoring network
- Lasso
Fuente:
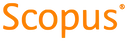
Tipo de documento:
Article
Estado:
Acceso restringido
Áreas de conocimiento:
- Optimización matemática
- Aprendizaje automático
- Meteorología
Áreas temáticas:
- Métodos informáticos especiales