Video processing inside embedded devices using SSD-Mobilenet to count mobility actors
Abstract:
The actual number of surveillance cameras and the different methods for counting vehicles originate the question: What is the best place to process video flows? This work performs the implementation of a counting system for mobility actors like cars, pedestrians, motorcycles, bicycles, buses, and trucks in the context of an Edge computing application using deep learning. However, the implementation of Deep Neural Networks for Object Detection in low-capacity embedded devices make it difficult to perform tasks that require high processing or must be carried out in real time. To solve this problem this study presents the analysis and implementation of different techniques based on the use of an additional hardware element as is the case of a Vision Processing Unit (VPU) in combination with methods that affect the resolution, bit rate, and time of video processing. For this purpose we consider the Mobilenet-SSD model with two approaches: a pre-trained model with known data sets and a trained model with images from our specific scenarios. The use of SSD-Mobilenet's model generates different results in terms of accuracy and time of video processing in the system. Results show that the use of an embedded device in combination with a VPU and video processing techniques reach 18.62 Frames per Second (FPS). Thus, video processing time is slightly superior (5.63 minutes) for a video of 5 minutes. Recall and precision values of 91% and 97% are reported in the best case (class car) for the vehicle counting system.
Año de publicación:
2019
Keywords:
- Raspberry PI
- Single Shot Detection
- MOBILENET
- Computer Vision
- Vision Processing Unit
Fuente:
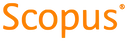
Tipo de documento:
Conference Object
Estado:
Acceso restringido
Áreas de conocimiento:
- Visión por computadora
- Ciencias de la computación
Áreas temáticas:
- Métodos informáticos especiales
- Ciencias de la computación
- Ingeniería y operaciones afines