Web mining based framework for solving usual problems in recommender systems. A case study for movies' recommendation
Abstract:
Endowing web systems with efficient and reliable recommendation procedures is being the target of intensive research in the last years. Numerous methods have been proposed to provide users with more and more effective recommendations, from the traditional collaborative filtering approaches to sophisticated web mining techniques, however some important drawbacks are still present in current recommender systems. Some works in the literature address these problems in an individual way. In this work, we propose a complete framework to deal jointly with some of the most important: scalability, sparsity, first rater and cold start problems. Although the framework is addressed to movies' recommendation and validated in this context it can be easily extended to other domains. It manages different pbkp_redictive models for making recommendations depending on specific situations. These models are induced by data mining algorithms using as input data both product and user attributes structured according to a particular domain ontology.
Año de publicación:
2016
Keywords:
- First-rater
- recommender systems
- sparsity
- associative classification
- semantic web mining
- Cold-start
Fuente:
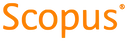
Tipo de documento:
Article
Estado:
Acceso restringido
Áreas de conocimiento:
- Minería de datos
- Ciencias de la computación
Áreas temáticas de Dewey:
- Métodos informáticos especiales
- Funcionamiento de bibliotecas y archivos
- Dirección general