Weibull Regression and Machine Learning Survival Models: Methodology, Comparison, and Application to Biomedical Data Related to Cardiac Surgery
Abstract:
In this article, we propose a comparative study between two models that can be used by researchers for the analysis of survival data: (i) the Weibull regression model and (ii) the random survival forest (RSF) model. The models are compared considering the error rate, the performance of the model through the Harrell C-index, and the identification of the relevant variables for survival prediction. A statistical analysis of a data set from the Heart Institute of the University of São Paulo, Brazil, has been carried out. In the study, the length of stay of patients undergoing cardiac surgery, within the operating room, was used as the response variable. The obtained results show that the RSF model has less error rate for the training and testing data sets, at 23.55% and 20.31%, respectively, than the Weibull model, which has an error rate of 23.82%. Regarding the Harrell C-index, we obtain the values 0.76, 0.79, and 0.76, for the RSF and Weibull models, respectively. After the selection procedure, the Weibull model contains variables associated with the type of protocol and type of patient being statistically significant at 5%. The RSF model chooses age, type of patient, and type of protocol as relevant variables for prediction. We employ the randomForestSRC package of the R software to perform our data analysis and computational experiments. The proposal that we present has many applications in biology and medicine, which are discussed in the conclusions of this work.
Año de publicación:
2023
Keywords:
- variable importance
- model diagnostics
- survival statistical analysis
- Harrell index
- non-normal regression
- statistical software
- binary trees
- random forest
- Weibull model
Fuente:
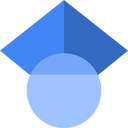
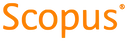
Tipo de documento:
Article
Estado:
Acceso abierto
Áreas de conocimiento:
- Estadísticas
- Aprendizaje automático
- Optimización matemática
Áreas temáticas de Dewey:
- Dibujo técnico, materiales peligrosos
- Medicina y salud
- Enfermedades

Objetivos de Desarrollo Sostenible:
- ODS 3: Salud y bienestar
- ODS 17: Alianzas para lograr los objetivos
- ODS 9: Industria, innovación e infraestructura
