Weight-Identification Model of Cattle Using Machine-Learning Techniques for Anomaly Detection
Abstract:
Cattle raising is an important economic activity, where livestock entrepreneurs keep track of their production and investment costs, to measure production and business profitability, based on cattle weighing. However, it is complicated for the farmer to detect if the animals they are weighing have gained the right weight. This paper proposes a framework to identify the fattening process, which can be used to detect anomalies in cattle weight-gain over time. This framework used records of animals raised and fattened at 'El Rosario' farm, located at the municipality of Monteriá (Córdoba-Colombia), to identify the fattening process. The performance of four machine-learning techniques to identify the ideal weight from real data was compared. The algorithms used were Decision Tree (DT), Gradient Boosting (GB), regression based on K-Nearest Neighbors (KNN), and Random Forest (RF). In addition, an outlier-detection process was performed to identify anomalous weights. In general, the results showed that the DT model was the one with the best performance with an average Mean Absolute Error (MAE) of 5.4 kg.
Año de publicación:
2021
Keywords:
- Machine learning
- Precision Livestock Farming
- Anomaly detection
- identification system
Fuente:
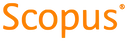
Tipo de documento:
Conference Object
Estado:
Acceso restringido
Áreas de conocimiento:
- Aprendizaje automático
- Ciencias de la computación
Áreas temáticas:
- Programación informática, programas, datos, seguridad
- Métodos informáticos especiales
- Agricultura y tecnologías afines