Weighted-PCA for unsupervised classification of cardiac arrhythmias
Abstract:
A method that improves the feature selection stage for non-supervised analysis of Holter ECG signals is presented. The method corresponds to WPCA approach developed mainly in two stages. First, the weighting of the feature set through a weight vector based on M-inner product as distance measure and a quadratic optimization function. The second one is the linear projection of weighted data using principal components. In the clustering stage, some procedures are considered: estimation of the number of groups, initialization of centroids and grouping by means a soft clustering algorithm. In order to decrease the procedure computational cost, segment analysis, grouping contiguous segments and establishing union and exclusion criteria per each cluster, is carried out. This work is focused to classify cardiac arrhythmias into 5 groups, according to the standard of the AAMI (ANSI/AAMI EC57:1998/2003). To validate the method, some recordings from MIT/BIH arrhythmia database are used. By employing the labels of each recording, the performance is assessed with supervised measures (Se = 90.1%, Sp = 98.9% y Cp = 97.4%), enhancing other works in the literature that do not take into account all heartbeat types. © 2010 IEEE.
Año de publicación:
2010
Keywords:
Fuente:
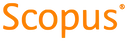
Tipo de documento:
Conference Object
Estado:
Acceso restringido
Áreas de conocimiento:
- Enfermedad cardiovascular
- Aprendizaje automático
- Ciencias de la computación
Áreas temáticas de Dewey:
- Enfermedades
- Fisiología humana
- Medicina y salud

Objetivos de Desarrollo Sostenible:
- ODS 3: Salud y bienestar
- ODS 17: Alianzas para lograr los objetivos
- ODS 9: Industria, innovación e infraestructura
