When Should We Use Linear Explanations?
Abstract:
The increasing interest in transparent and fair AI systems has propelled the research in explainable AI (XAI). One of the main research lines in XAI is post-hoc explainability, the task of explaining the logic of an already deployed black-box model. This is usually achieved by learning an interpretable surrogate function that approximates the black box. Among the existing explanation paradigms, local linear explanations are one of the most popular due to their simplicity and fidelity. Despite their advantages, linear surrogates may not always be the most adapted method to produce reliable, i.e., unambiguous and faithful explanations. Hence, this paper introduces Adapted Post-hoc Explanations (APE), a novel method that characterizes the decision boundary of a black-box classifier and identifies when a linear model constitutes a reliable explanation. Besides, characterizing the black-box frontier allows us to provide complementary counterfactual explanations. Our experimental evaluation shows that APE identifies accurately the situations where linear surrogates are suitable while also providing meaningful counterfactual explanations.
Año de publicación:
2022
Keywords:
- Explainability
- rule-based explanations
- interpretability
- linear explanations
- counterfactual explanations
Fuente:
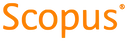
Tipo de documento:
Conference Object
Estado:
Acceso restringido
Áreas de conocimiento:
- Filosofía de la ciencia
- Aprendizaje automático
Áreas temáticas de Dewey:
- Sociología y antropología
- Psicología diferencial y del desarrollo
- Procesos mentales conscientes e inteligencia