White blood cell classification and counting using convolutional neural network
Abstract:
Establishing an accurate count and classification of leukocytes commonly known as WBC (white blood cells) is crucial in the assessment and detection of illness of an individual, which involves complications on the immune system that leads to various types of diseases including infections, anemia, leukemia, cancer, AIDS (Acquired Immune Deficiency Syndrome) etc. The two widely used methods to count WBC is with the use of hematology analyzer and manual counting. Currently, in the age of modernization there has been numerous research in the field of image processing incorporated with various segmentation and classification techniques to be able to generate alternatives for WBC classification and counting. However, the accuracy of these existing methods could still be improved. Thus, in this paper we proposed a new method that could segment various types of WBCs: monocytes, lymphocytes, eosinophils, basophils, and neutrophils from a microscopic blood image using HSV (Hue, Saturation, Value) saturation component with blob analysis for segmentation and incorporate CNN (Convolutional Neural Network) for counting which in turn generates more accurate results.
Año de publicación:
2018
Keywords:
- Leukocytes
- HSV image processing
- Convolutional neural network
- white blood cells
- blob analysis
Fuente:
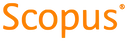
Tipo de documento:
Conference Object
Estado:
Acceso restringido
Áreas de conocimiento:
- Inmunología
- Aprendizaje automático
- Ciencias de la computación
Áreas temáticas:
- Ciencias de la computación
- Métodos informáticos especiales
- Medicina y salud