Wind Turbine Main Bearing Failure Pbkp_rediction using a Hybrid Neural Network
Abstract:
Energy is necessary for economic growth and improved well-being, but it poses a great challenge to be generated without increasing costs and avoiding pollution. A viable option is wind energy because it is a clean and renewable. However, continuous monitoring and maintenance of wind turbines is required for the further development of wind farms. Main bearing failures were identified by the European Academy of Wind Energy as a critical issue in terms of increasing the availability and reliability of wind turbines. In this work, it is proposed a hybrid neural network for main bearing failure prognosis. This network consists of a two-dimensional convolutional neural network (to extract spatial-temporal characteristics from the data) sequentially connected with a long short-term memory network (to learn sequence patterns) to pbkp_redict the slow-speed shaft temperature (the closest temperature to the main bearing). The mean square error between its real measurement and its pbkp_rediction gives a failure indicator. When it is greater than a defined threshold, then an alarm is triggered and gives the maintenance staff time to check the component. The advantage of this strategy is that it does not need faulty data to be trained, since it is based on a normality model, that is, it is trained with a single class of data (healthy) and does not require incurring high costs per acquisition of new sensors since SCADA data is used (which comes in all industrial size turbine). The results show that the use of a hybrid network can identify failures around four months before a fatal failure occurs.
Año de publicación:
2022
Keywords:
Fuente:
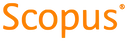
Tipo de documento:
Conference Object
Estado:
Acceso abierto
Áreas de conocimiento:
- Ingeniería mecánica
- Red neuronal artificial
Áreas temáticas:
- Física aplicada
- Métodos informáticos especiales