Wind Turbine Multi-Fault Detection and Classification using Machine Learning Techniques
Abstract:
In the last years, there has been an increase in the number of places where wind power is exploited to generate energy through the use of wind turbines (WTs). In this sense, with the increasing of installation capacity of wind power, advanced fault detection (FD), and classification strategies have become crucial to accomplish the required levels of reliability and availability. In this work, the WT we consider is subject to different types of faults on actuators and sensors and only is increased the sampling frequency in the already available (in all commercial WTs) sensors of the Supervisory Control and Data Acquisition (SCADA) system to develop the strategies. Support vector machine (SVM) and Extreme Gradient Boosting (XGBoost) algorithms are used to classify the different faults. One of the challenges of this research is that the disturbance signal (the wind) is unknown. A generic 5 MW WT benchmark model was used to evaluate the proposed approaches and the comparative results demonstrate that the proposed methods can significantly enhance the classification performance.
Año de publicación:
2021
Keywords:
- Wind turbine
- data augmentation
- Multi-fault classification
- S V M
- Scada
- XGBoost
Fuente:
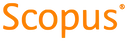
Tipo de documento:
Conference Object
Estado:
Acceso restringido
Áreas de conocimiento:
- Software
- Aprendizaje automático
Áreas temáticas:
- Física aplicada
- Métodos informáticos especiales
- Instrumentos de precisión y otros dispositivos