Wind turbine main bearing condition monitoring via convolutional autoencoder neural networks
Abstract:
The rapid growth of the energy capacity generated by wind turbines, as well as their size, creates new challenges in terms of operation and maintenance. Main bearing failures have been identified as one of the main and most critical issues in terms of increasing the availability and reliability of wind turbines. Therefore, pbkp_redicting wind turbine main bearing failures has become a technical challenge and an economically important issue. In this manuscript it is established a fault prognosis procedure to forecast main bearing failures several months beforehand to help wind turbine operators plan maintenance in advance. Reducing downtime is one of the main goals in wind energy industry since it lets addressing its energy loss impact. The main contributions of this work are described below: i) the prognosis is carried out by using only SCADA data, which is accessible in all commercial wind turbines; therefore, no additional sensors designed for a determined purpose need to be installed; ii) it is a semi-supervised approach, thus it only requires healthy data to be collected; iii) it is based only on external variables and one representative temperature close to the subsystem under diagnosis, thus avoiding data contamination; iv) it accomplishes the prognosis (several months beforehand) of the main bearing fault; and v) the performance and validity of the proposed strategy are demonstrated on a real underproduction wind turbine.
Año de publicación:
2021
Keywords:
- Real SCADA data
- Fault prognosis
- Main bearing
- Neural networks
- Wind turbine
- Normality Model
- Convolutional autoencoder
Fuente:
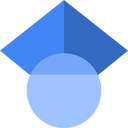
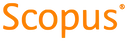
Tipo de documento:
Conference Object
Estado:
Acceso restringido
Áreas de conocimiento:
- Ciencias de la computación
- Aprendizaje automático
Áreas temáticas:
- Métodos informáticos especiales
- Física aplicada