Woody cover fractions in African Savannas from landsat and high-resolution imagery
Abstract:
The challenge of mapping savanna vegetation has limited our understanding of the factors that shape these ecosystems at large scales. We tested seven methods for mapping savanna woody cover (trees and shrubs; WC) across 12 protected areas (PAs) in eastern Africa using Landsat 8 imagery. Because we wanted a method viable for mapping across the often-limited Landsat historical archive, we limited ourselves to three images: one each from the wet, dry, and transition (halfway between wet and dry) seasons. Models were trained and tested using 1,330 WC reference points and the variance explained by cross validation (VEcv) accuracy metric. Of the methods we tested, RF significantly (p < 0.001) outperformed the others with the best models in nine PAs scoring over 75% (range of 34.5%-91.1%). RF models trained using data from all the PAs and tested in the individual PAs significantly (p < 0.001) outperformed their single-PA-derived counterparts (67.7 ± 23.3% versus 30.5 ± 27.4%). We also found that while the transition image appears to be critical to mapping WC and the wet season image should be avoided, no single season or seasonal combination significantly outperformed all the others, allowing some flexibility in image selection. Our findings show that with proper sampling of landscape heterogeneity, even with limited imagery, accurate maps of savanna WC are possible and could catalyze discoveries in this crucial biome.
Año de publicación:
2020
Keywords:
- Landsat
- random forest
- regression
- Spectral unmixing
- GOOGLE EARTH ENGINE
- ÁFRICA
- Savanna ecology
- Woody cover maps
Fuente:
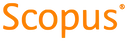
Tipo de documento:
Article
Estado:
Acceso abierto
Áreas de conocimiento:
- Sensores remotos
- Geografía
Áreas temáticas:
- Agricultura y tecnologías afines