Word-embedding-based model to improve the customer’ shopping experience
Abstract:
The present work experiments with different techniques in order to build a pbkp_redictive model that can be able to solve the Kaggle’s competition called “Home Depot Product Search Relevance” which is a supervised machine learning problem. We used several Natural Language Processing methods like tokenization, lemmatization, extracting stop words and Mikolov’s word2vec among others. We combine these techniques for feature extraction and utilize the Random Forest algorithm for building the regression model. Finally, we use the R open-source software for conducting the experiment. The results indicate that the use of word embeddings gave the best pbkp_redictor model and can be a useful technique for many NLP applications.
Año de publicación:
2019
Keywords:
- Machine learning
- Regression model
- Word embeddings
- NLP
Fuente:
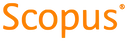
Tipo de documento:
Article
Estado:
Acceso restringido
Áreas de conocimiento:
- Marketing
- Inteligencia artificial
- Ciencias de la computación
Áreas temáticas:
- Funcionamiento de bibliotecas y archivos