A feature-based performance analysis in evolutionary multiobjective optimization
Abstract:
This paper fundamentally investigates the performance of evolutionary multiobjective optimization (EMO) algorithms for computationally hard 0–1 combinatorial optimization, where a strict theoretical analysis is generally out of reach due to the high complexity of the underlying problem. Based on the examination of problem features from a multiobjective perspective, we improve the understanding of the efficiency of a simple dominance-based EMO algorithm with unbounded archive for multiobjective NK-landscapes with correlated objective values. More particularly, we adopt a statistical approach, based on simple and multiple linear regression analysis, to enquire the expected running time of global SEMO with restart for identifying a (1 + ε)−approximation of the Pareto set for small-size enumerable instances. Our analysis provides further insights on the EMO search behavior and on the most important features that characterize the difficulty of an instance for this class of problems and algorithms.
Año de publicación:
2015
Keywords:
Fuente:
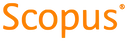
Tipo de documento:
Conference Object
Estado:
Acceso restringido
Áreas de conocimiento:
- Optimización matemática
- Optimización matemática
- Control óptimo
Áreas temáticas:
- Programación informática, programas, datos, seguridad
- Biología
- Dirección general